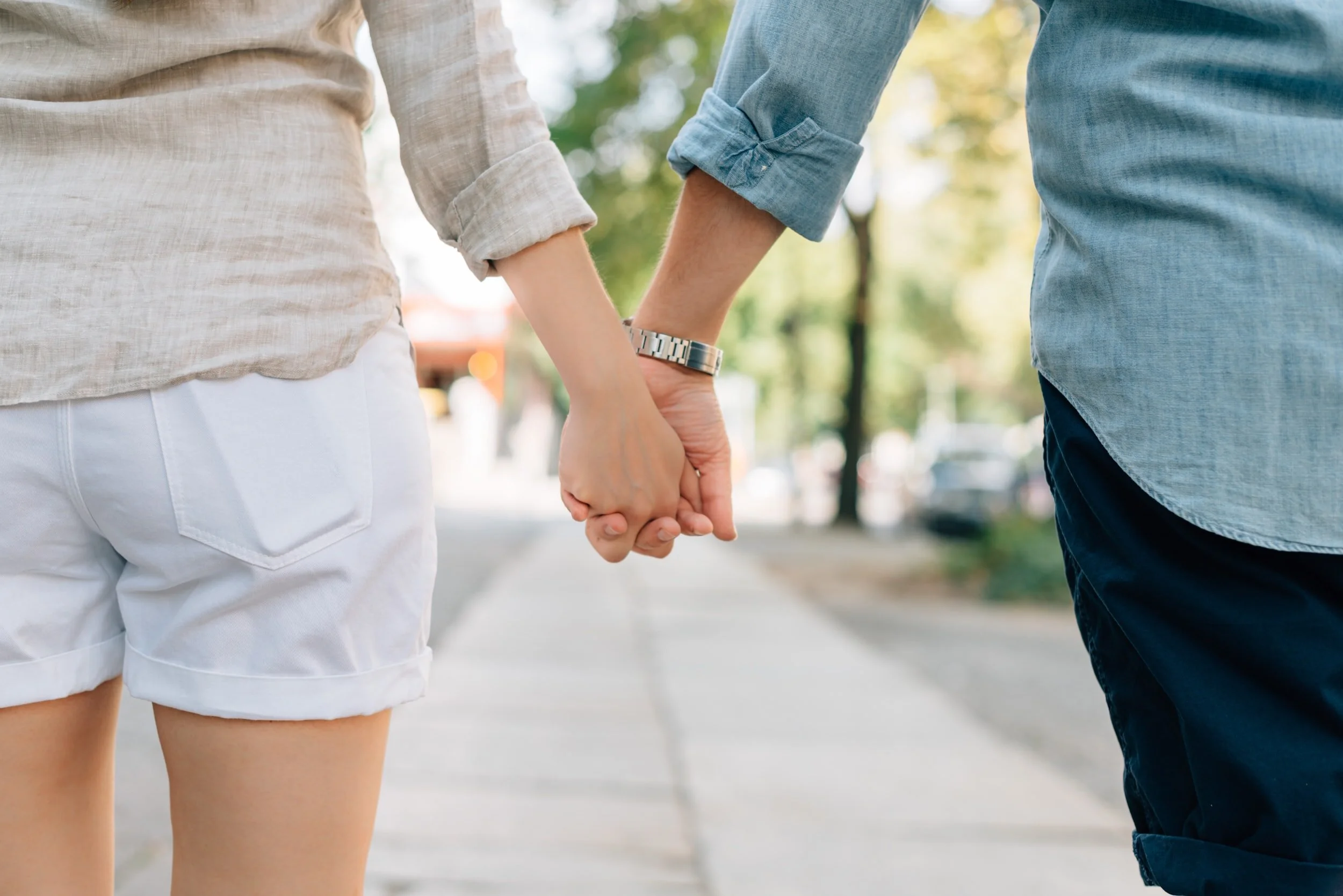
HOPE: A multi-site research study seeking to find explanations for miscarriage.
HOPE, Harnessing multiple Opportunities for Pregnancy loss Exploration, is a national research study that seeks to find explanations of pregnancy loss and predict future pregnancy outcomes.
While more than 3000 human genes are conserved and likely essential for early development remarkably little is known about their contribution to recurrent pregnancy loss and current genetic databases are essentially devoid of these entries. We believe that through genetic and molecular profiling of trios (mom-dad-past pregnancy), we will be able to confirm which genes contribute to pregnancy loss and discover novel variants in genes and gene pathways essential to human development.
Aim 1
Build a national registry and biobank of patients who have experienced one or more pregnancy loss, that can be used to accelerate discovery and identify genetic and clinical predictors of future pregnancy outcomes.
Aim 2
Analyze whole genome sequences of recurrent pregnancy loss trios.
Aim 3
Use molecular testing to clarify how gene expression results in biological pathways/mechanisms that lead to miscarriage
Aim 4
Engage in machine learning to improve prediction of pregnancy loss.
The Science
We aim to understand what biologic factors (genes or chromosome regions and molecular profiles) are associated with unexplained pregnancy loss. The human genome contains 3 billion letters (base pairs) that make up approximately 100,000 genes. Performing exploratory genetic testing on multiple members of the same family allows us to better determine which genes and gene changes (mutations) are associated with diseases and which are associated with health.
A trio analysis is a type of genetic analysis where 2 parents and a pregnancy (or baby) are analyzed together to discover inheritance and gene changes that cause the disease in the affected pregnancy. The combination of medical history and genetic information helps us understand the relationship with new genetic markers with unexplained medical conditions such as infertility, miscarriage and pregnancy loss. The novel informatics approaches used in this study will uncover genes and gene pathways previously unrecognized with standard genetic testing approaches. The genetic markers found in this research will help us understand the causes of recurrent pregnancy loss and eventually lead to new tests and treatments for families with the diagnosis of unexplained pregnancy loss.
Machine Learning
Machine learning is a powerful computational technique for recognizing and identifying patterns hidden in large amounts of complex data. With the advent of high-throughput sequencing and lower cost of computation, machine learning is becoming more popular to exploit those data for pinpointing the molecular bases of complex human diseases, paving the way to precision medicine for pre-screening, diagnosis and treatment. We plan to use novel machine learning approaches to discover risk genes and pathways underpinning the various presentations (gestational ages, number of losses, obstetric history) of recurrent pregnancy loss and to predict future pregnancy loss.
Collaboration
Finally, we hope to increase worldwide collaboration on pregnancy loss research through making de-identified and curated data publicly available by creating a repository of genotype-phenotype associations found in this study. No personal or private information will be shared.
There are currently few resources available to clinical geneticists, patients or researchers which are effective at gathering the relevant genetic information for recurrent pregnancy loss and pregnancy loss in general. Searching NCBI GEO for ‘miscarriage’, ‘recurrent pregnancy loss’, and equivalent terms returns only a handful of studies, each with only a few samples. Thus, there is a huge unmet need to generate and share high quality, curated data to allow clinicians and researchers to better inform diagnostics and therapeutics for RPL. We will develop a new database that combines clinical, imaging, and molecular phenotyping with genotype so that the presentations rare to a single center can be aggregated and the prenatal diagnostic acumen, including for demise, be enhanced. We will build on our extensive previous experience in prenatal databases supported by the March of Dimes to create the “prenatal OMIM,” focused on the prenatal phenotypic presentation of Mendelian conditions, including those that cause fetal loss and genes associated with those phenotypes, which will be further enhanced by multiomics data.